Research
PU-GCN: Point Cloud Upsampling using Graph Convolutional Networks

Guocheng Qian, Abdulellah Abualshour, Guohao Li, Ali Thabet, Bernard Ghanem
King Abdullah University of Science and Technology (KAUST), Saudi Arabia
CVPR'21
The effectiveness of learning-based point cloud upsampling pipelines heavily relies on the upsampling modules and feature extractors used therein. For the point upsampling module, we propose a novel model called NodeShuffle, which uses a Graph Convolutional Network (GCN) to better encode local point information from point neighborhoods. NodeShuffle is versatile and can be incorporated into any point cloud upsampling pipeline. Extensive experiments show how NodeShuffle consistently improves state-ofthe-art upsampling methods. For feature extraction, we also propose a new multi-scale point feature extractor, called Inception DenseGCN. By aggregating features at multiple scales, this feature extractor enables further performance gain in the final upsampled point clouds. We combine Inception DenseGCN with NodeShuffle into a new point upsampling pipeline called PU-GCN. PU-GCN sets new stateof-art performance with much fewer parameters and more efficient inference.
AdvPC: Transferable Adversarial Perturbations on 3D Point Clouds

Abdullah Jamal Hamdi, Sara Rojas Martinez, Ali Thabet, Bernard Ghanem
King Abdullah University of Science and Technology (KAUST), Saudi Arabia
ECCV'20
Deep neural networks are vulnerable to adversarial attacks, in which imperceptible perturbations to their input lead to erroneous network predictions. This phenomenon has been extensively studied in the image domain, and has only recently been extended to 3D point clouds. In this work, we present novel data-driven adversarial attacks against 3D point cloud networks. We aim to address the following problems in current 3D point cloud adversarial attacks: they do not transfer well between different networks, and they are easy to defend against via simple statistical methods. To this extent, we develop a new point cloud attack (dubbed AdvPC) that exploits the input data distribution by adding an adversarial loss after Auto-Encoder reconstruction to the objective it optimizes. AdvPC leads to perturbations that are resilient against current defenses while remaining highly transferable compared to state-of-the-art attacks. We test AdvPC using four popular point cloud networks: PointNet, PointNet++ (MSG and SSG), and DGCNN. Our proposed attack increases the attack success rate by up to 40 % on transferred attacks to unseen networks (transferability), while maintaining a high success rate on the attacked network. AdvPC also increases the ability to break defenses by up to 38 % as compared to other baselines on ModelNet40 data.
Gabor Layers Enhance Network Robustness
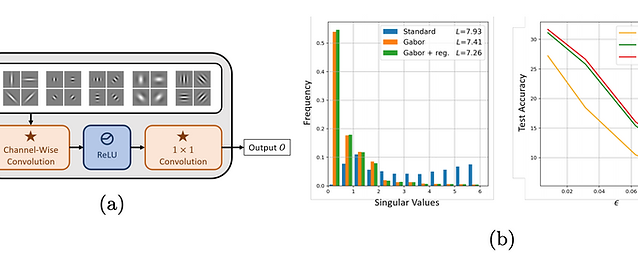
Juan C. Pérez*, Motasem Alfarra**, Guillaume Jeanneret*, Adel Bibi**, Ali Thabet**, Bernard Ghanem**, Pablo Arbeláez*
*Universidad de los Andes, Colombia
**King Abdullah University of Science and Technology (KAUST), Saudi Arabia
ECCV'20
We revisit the benefits of merging classical vision concepts with deep learning models. In particular, we explore the effect on robustness against adversarial attacks of replacing the first layers of various deep architectures with Gabor layers, i.e. convolutional layers with filters that are based on learnable Gabor parameters. We observe that architectures enhanced with Gabor layers gain a consistent boost in robustness over regular models and preserve high generalizing test performance, even though these layers come at a negligible increase in the number of parameters. We then exploit the closed form expression of Gabor filters to derive an expression for a Lipschitz constant of such filters, and harness this theoretical result to develop a regularizer we use during training to further enhance network robustness. We conduct extensive experiments with various architectures (LeNet, AlexNet, VGG16 and WideResNet) on several datasets (MNIST, SVHN, CIFAR10 and CIFAR100) and demonstrate large empirical robustness gains. Furthermore, we experimentally show how our regularizer provides consistent robustness improvements.
Self-Supervised Learning of Local Features in 3D Point Clouds
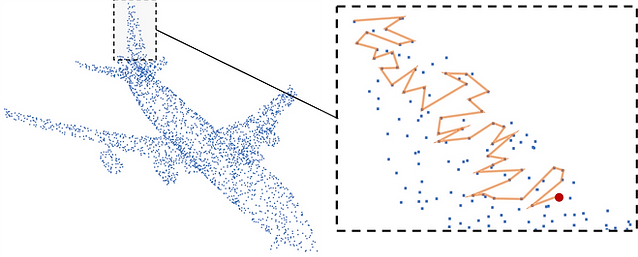
Ali Thabet, Humam Alwassel, Bernard Ghanem
King Abdullah University of Science and Technology (KAUST), Saudi Arabia
CVPRW'20
We present a self-supervised task on point clouds, in order to learn meaningful point-wise features that encode local structure around each point. Our self-supervised network, operates directly on unstructured/unordered point clouds. Using a multi-layer RNN, our architecture predicts the next point in a point sequence created by a popular and fast Space Filling Curve, the Morton-order curve. The final RNN state (coined Morton feature) is versatile and can be used in generic 3D tasks on point clouds. Our experiments show how our self-supervised task results in features that are useful for 3D segmentation tasks, and generalize well between datasets. We show how Morton features can be used to significantly improve performance (3% for 2 popular algorithms) in semantic segmentation of point clouds on the challenging and large-scale S3DIS dataset. We also show how our self-supervised network pretrained on S3DIS transfers well to another large-scale dataset, vKITTI, leading to 11% improvement.
SGAS: Sequential Greedy Architecture Search
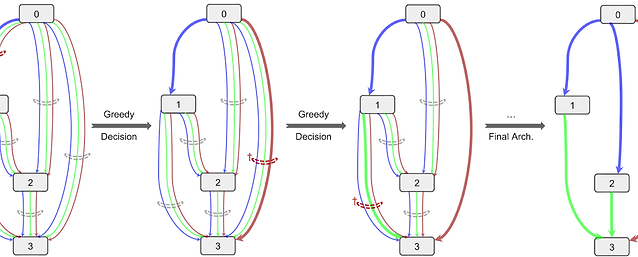
Guohao Li, Guocheng Qian, Itzel C. Delgadillo, Matthias Müller, Ali Thabet, Bernard Ghanem
King Abdullah University of Science and Technology (KAUST), Saudi Arabia
CVPR'20
Architecture design has become a crucial component of successful deep learning. Recent progress in automatic neural architecture search (NAS) shows a lot of promise. However, discovered architectures often fail to generalize in the final evaluation. Architectures with a higher validation accuracy during the search phase may perform worse in the evaluation. Aiming to alleviate this common issue, we introduce sequential greedy architecture search (SGAS), an efficient method for neural architecture search. By dividing the search procedure into sub-problems, SGAS chooses and prunes candidate operations in a greedy fashion. We apply SGAS to search architectures for Convolutional Neural Networks (CNN) and Graph Convolutional Networks (GCN). Extensive experiments show that SGAS is able to find state-of-the-art architectures for tasks such as image classification, point cloud classification and node classification in protein-protein interaction graphs with minimal computational cost
G-TAD: Sub-Graph Localization for Temporal Action Detection
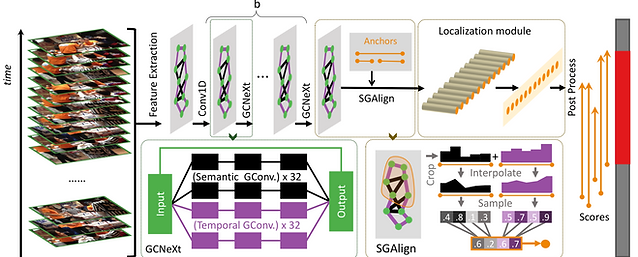
Mengmeng Xu, Chen Zhao, David S. Rojas, Ali Thabet, Bernard Ghanem
King Abdullah University of Science and Technology (KAUST), Saudi Arabia
CVPR'20
Temporal action detection is a fundamental yet challenging task in video understanding. Video context is a critical cue to effectively detect actions, but current works mainly focus on temporal context, while neglecting semantic con-text as well as other important context properties. In this work, we propose a graph convolutional network (GCN) model to adaptively incorporate multi-level semantic context into video features and cast temporal action detection as a sub-graph localization problem. Specifically, we formulate video snippets as graph nodes, snippet-snippet cor-relations as edges, and actions associated with context as target sub-graphs. With graph convolution as the basic operation, we design a GCN block called GCNeXt, which learns the features of each node by aggregating its context and dynamically updates the edges in the graph. To localize each sub-graph, we also design a SGAlign layer to embed each sub-graph into the Euclidean space. Extensive experiments show that G-TAD is capable of finding effective video context without extra supervision and achieves state-of-the-art performance on two detection benchmarks. On ActityNet-1.3, we obtain an average mAP of 34.09%; on THUMOS14, we obtain 40.16% in mAP@0.5, beating all the other one-stage methods.
Can GCNs Go as Deep as CNNs?
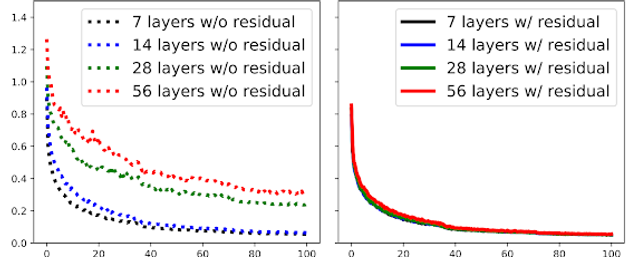
Guohao Li, Matthias Müller, Ali Thabet, Bernard Ghanem
King Abdullah University of Science and Technology (KAUST), Saudi Arabia
ICCV'19 - Oral
Convolutional Neural Networks (CNNs) achieve impressive results in a wide variety of fields. Their success benefited from a massive boost with the ability to train very deep CNN models. Despite their positive results, CNNs fail to properly address problems with non-Euclidean data. To overcome this challenge, Graph Convolutional Networks (GCNs) build graphs to represent non-Euclidean data, and borrow concepts from CNNs and apply them to train these models. GCNs show promising results, but they are limited to very shallow models due to the vanishing gradient problem. As a result most state-of-the-art GCN algorithms are no deeper than 3 or 4 layers. In this work, we present new ways to successfully train very deep GCNs. We borrow concepts from CNNs, mainly residual/dense connections and dilated convolutions, and adapt them to GCN architectures. Through extensive experiments, we show the positive effect of these deep GCN frameworks. Finally, we use these new concepts to build a very deep 56-layer GCN, and show how it significantly boosts performance (+3.7% mIoU over state-of-the-art) in the task of point cloud semantic segmentation.